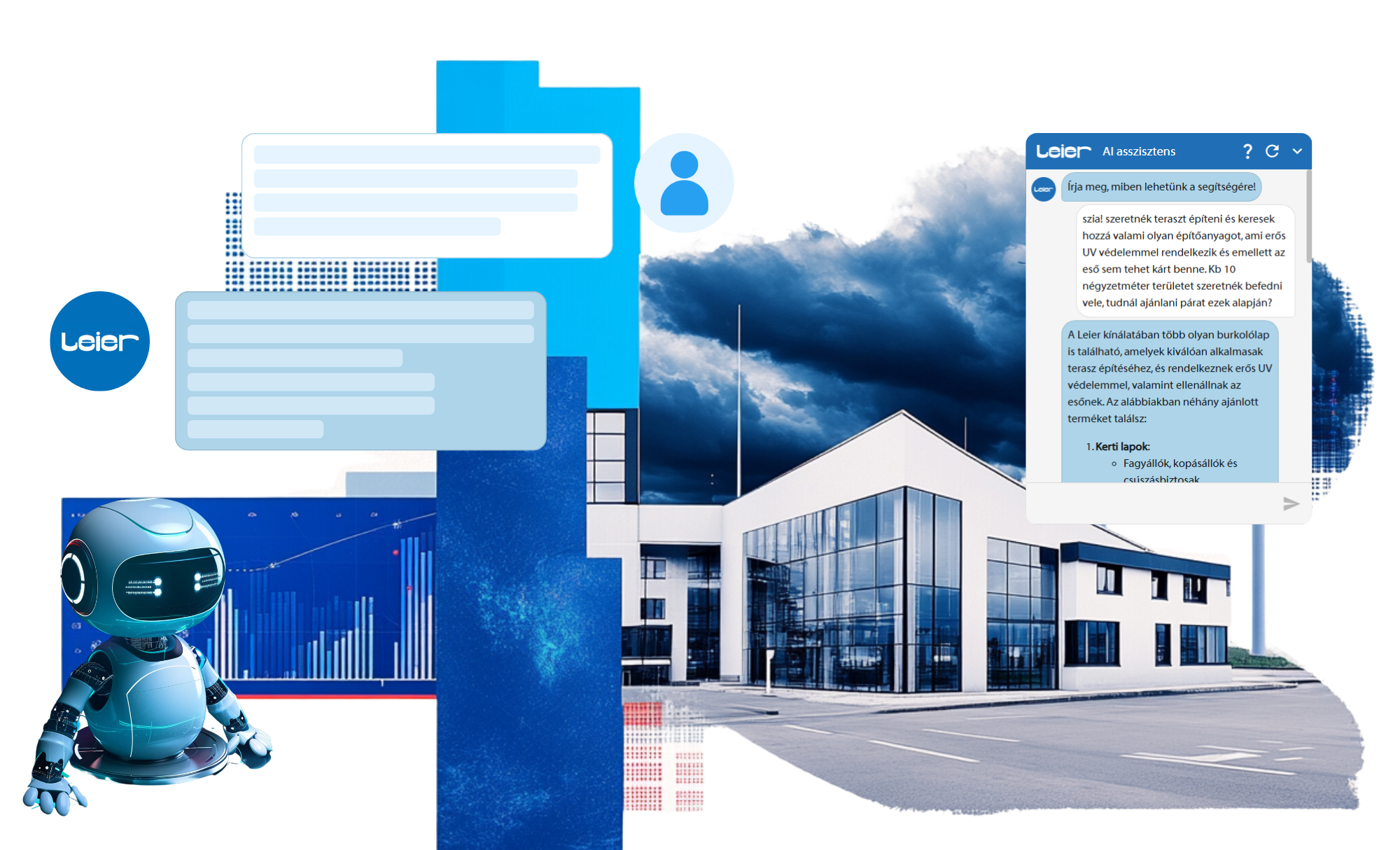
Közös fejlesztés, közös siker - Leier AI Asszisztens
„Az AI nem cél, hanem eszköz” – Interjú Somogyi Imrével, az Attrecto ügyvezetőjével
Mi vezetett az AI asszisztens fejlesztéséhez?
Amikor elkezdtük az együttműködést, egyértelmű volt, hogy nem csupán egy újabb technológiai fejlesztésről lesz szó. Közösen azonosítottunk egy olyan üzleti kihívást, amely jelentős hatással volt az értékesítési csapat hatékonyságára és az ügyfélélményre is, és onnan már egyértelmű volt, hogy a leghatékonyabban ezzel tudjuk segíteni a Leiert.
Milyen problémát sikerült azonosítani, és hogyan közelítettétek meg a megoldást?
A legnagyobb felismerés az volt, hogy az értékesítők és ügyfélszolgálati munkatársak idejük jelentős részét ismétlődő, viszont olykor nagyon részletekbe menő kérdések megválaszolásával töltötték – például: mi a hőátbocsátási tényezője a 25/18-as durisol falazóelemnek, melyik nyílásáthidalót használják a 30-as téglához, vagy éppen hány térkő szükséges egy 500 nm-es kertbe. Ilyen részleteket 6000+ cikk esetén lehetetlen fejben tartani, a kollégáknak rengeteg ideje ment el keresgéléssel, számolgatással.
Arra törekedtünk, hogy ezt a terhet csökkentsük, és lehetőséget teremtsünk a kollégáknak arra, hogy magasabb hozzáadott értékű tevékenységekre koncentrálhassanak. Az AI-asszisztens nem kiváltja a munkájukat, hanem megerősíti és támogatja őket a személyes kapcsolatok építésében, a szaktanácsadásban, valamint a komplex problémák megoldásában.
Mitől vált különlegessé ez a fejlesztés?
Az, ahogyan a technológia és az üzleti igények találkoztak. Végig arra törekedtünk, hogy ne csupán egy terméket szabjunk testre, hanem valódi választ adjunk a Leier napi működési kihívásaira. Olyan AI asszisztenst hoztunk létre, amely természetes párbeszédet tesz lehetővé, miközben szakmailag pontos válaszokat ad. A heti egyeztetések, az iteratív fejlesztés, a gyors visszacsatolás és a Leier szakértőinek aktív bevonódása lehetővé tették, hogy folyamatosan finomhangoljuk az asszisztenst. Ez a közös munka tette igazán értékessé és működőképessé a rendszert.
Mi az, amire különösen büszke vagy a projekt kapcsán?
Több dolog is eszembe jut. Egyrészt arra, hogy nem csupán egy informatikai terméket adtunk át, hanem a Leierrel közösen egy teljesen új technológiai irányt vezettünk be, amely kézzelfogható üzleti értéket teremtett. Másrészt különösen büszke vagyok arra a partnerségre és bizalmi légkörre, amely a közös munka során kialakult – ez alapozta meg a projekt sikerét.
A megvalósult AI asszisztens az esetek több mint 85%-ában önállóan, emberi beavatkozás nélkül kezeli a beérkező megkereséseket. Átlagos válaszideje 3,61 másodperc, ami alatt átvizsgálja a Leier előzetesen strukturált, ~27.000 oldalnyi információt tartalmazó dokumentumtárát, azonosítja a releváns információkat, kiértékeli őket, és megfogalmazza a választ – mindezt az esetek 99%-ában ebben a sebességben (P99 latency). Ha a válasz nem igényel dokumentumalapú keresést, az asszisztens akár 0,4 másodpercen belül is képes megkezdeni a válaszadást.
Ahogy Turbók Balázs, a Leier értékesítési vezetője is megfogalmazta: „Maximálisan figyelembe vettük az igényeiket.” Ez a hozzáállás, valamint a folyamatos együttműködés és rugalmasság legalább akkora értéket képvisel, mint maguk az elért eredmények.
Sokan tartanak attól, hogy az AI elveszi a munkát. Ti hogyan látjátok ezt?
Mi nem úgy tekintünk az AI-ra, mint ami kiváltja az embert, hanem mint ami kiegészíti és támogatja. Olyan feladatokat, esetleg olyan mennyiségű vagy minőségű feladatokat, amire eddig a technológia vagy az időnk korlátossága nem adott lehetőséget. Az a cél, hogy az emberek több időt és energiát tudjanak fordítani arra, amiben igazán jók, és ezt az AI-nak köszönhetően meg is tehetik. A legjobb eredmények akkor születnek, ha a technológiát nem az ember helyett, hanem az emberrel együtt alkalmazzuk.
Milyen szerepe volt az adatbiztonságnak a fejlesztés során?
Ez kezdettől fogva kiemelt szempont volt. A rendszer a Microsoft Azure környezetben fut, és hibrid adatkezelési modellt alkalmaz, azaz csak a legszükségesebb adatok kerülnek a felhőbe, így biztosítva a magas szintű adatvédelmet. Az ügyfél és a felhasználók bizalmát csak úgy tudjuk megőrizni, ha a technológia mögött stabil, átlátható és biztonságos háttér van.
Mi a következő lépés?
A fejlesztés nem ér véget az asszisztens bevezetésével. Már dolgozunk a funkcionalitás bővítésén, hogy más üzleti folyamatokat is támogathassunk. Hosszú távon az a célunk, hogy az AI természetesen beépüljön a vállalat napi működésébe, és mindig az aktuális üzleti célokat segítse.
Mit üzennél azoknak a vállalatoknak, akik még csak most gondolkodnak AI-megoldások bevezetésén?
Azt, hogy ne a technológiával kezdjék, hanem az üzleti problémával. Az AI nem cél, hanem eszköz – eszköz ahhoz, hogy jobb döntéseket hozzunk, értékesebb munkát végezzünk, és jobb szolgáltatást nyújtsunk. A megfelelő partnerrel együtt a technológia valódi értéket tud teremteni. Ha ez a szemlélet megvan, onnan már csak egy lépés a valódi innováció. A siker kulcsa a közös gondolkodásban és az együttműködésben rejlik.
Collaborative Development, Shared Success - Leier AI Assistant
“AI is not a goal, but a tool” – Interview with Imre Somogyi, CEO of Attrecto
What led to the development of the AI assistant?
When we started the collaboration, it was clear that this wouldn’t just be another technological development. Together, we identified a business challenge that had a significant impact on both the sales team’s efficiency and the customer experience, and from there it was obvious that this was the most effective way we could help Leier.
What problem did you manage to identify, and how did you approach the solution?
The biggest realisation was that sales representatives and customer service staff were spending a significant portion of their time answering repetitive, yet often very detailed questions – for example: what is the thermal conductivity coefficient of the 25/18 durisol masonry element, which lintel should be used with 30cm brick, or exactly how many paving stones are needed for a 500 square metre garden. With 6000+ products, it’s impossible to keep such details in your head, and colleagues were spending enormous amounts of time searching and calculating.
We strived to reduce this burden and create opportunities for colleagues to concentrate on higher value-added activities. The AI assistant doesn’t replace their work, but strengthens and supports them in building personal relationships, providing expert consultation, and solving complex problems.
What made this development special?
How technology and business needs came together. Throughout, we strived not just to customise a product, but to provide a real answer to Leier’s daily operational challenges. We created an AI assistant that enables natural dialogue while providing professionally accurate answers. The weekly meetings, iterative development, quick feedback, and active involvement of Leier’s experts allowed us to continuously fine-tune the assistant. This collaborative work is what made the system truly valuable and functional.
What are you particularly proud of regarding this project?
A few things come to mind. First, I’m proud that we didn’t just deliver a digital product – we introduced an entirely new technological direction together with Leier, one that brings tangible business value. Second, I’m especially proud of the partnership and the mutual trust that developed throughout the project. That strong foundation of collaboration was key to the project’s success.
The AI assistant we implemented now handles over 85% of incoming inquiries independently, without the need for human intervention. Its average response time is 3.61 seconds – during which it scans Leier’s pre-processed document repository (across ~27,000 pages), identifies relevant information, evaluates it, and formulates an accurate answer. This performance is sustained in 99% of cases (P99 latency). When the assistant can respond based on its internal knowledge alone, it can start delivering an answer in as little as 0.4 seconds.
As Balázs Turbók, Leier’s Head of Sales, put it: “We made sure their needs were fully taken into account.” That mindset — along with continuous collaboration and flexibility — proved to be just as valuable as the results themselves.
Many people fear that AI will take away jobs. How do you see this?
We don’t view AI as something that replaces humans, but as something that complements and supports them. Tasks, or perhaps such quantities or qualities of tasks, that previously our technology or time limitations didn’t allow for. The goal is for people to be able to spend more time and energy on what they’re really good at, and thanks to AI, they can do just that. The best results are achieved when we apply technology not instead of humans, but together with humans.
What role did data security play during development?
This was a priority consideration from the beginning. The system runs in the Microsoft Azure environment and uses a hybrid data management model, meaning only the most necessary data goes to the cloud, thus ensuring high-level data protection. We can only maintain the trust of clients and users if there’s a stable, transparent, and secure background behind the technology.
What's the next step?
Development doesn’t end with the introduction of the assistant. We’re already working on expanding functionality to support other business processes as well. Our long-term goal is for AI to naturally integrate into the company’s daily operations and always support current business objectives.
What would you tell companies that are just now thinking about implementing AI solutions?
That they shouldn’t start with technology, but with the business problem. AI is not a goal, but a tool – a tool to make better decisions, do more valuable work, and provide better service. With the right partner, technology can create real value, and with this mindset it’s just one step to real innovation. The key to success lies in thinking together and collaboration.